 |  | 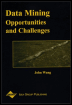 | | Chapter XV - Data Mining in Health Care Applications | Data Mining: Opportunities and Challenges | by John Wang (ed) | | Idea Group Publishing 2003 | | | | Brought to you by Team-Fly | | | HOW THE PLATFORM CAN BE USED CHIN technology would enable health care constituents to electronically create, maintain, and transform medical data via internal organizational and network-wide databases. Medical information can be housed in several tables, such as patient info, employer info, dependent info, medical records, physician info, etc. Attributes describing each entity with appropriate relationships would be established. These databases can, then, feed into a data warehouse with data marts for each CHIN constituent. These data marts can be used to manage specialized processing by individual CHIN organization. Data from the CHIN overarching database is extractable to facilitate data-mining processes. Data mining often follows one of several methods: -
Predictive modeling includes classification and regression techniques that are used to forecast unknown outcomes or variables; -
Clustering establishes clusters (based on an analogous statistic) in order to compare historical data to analyze new data; -
Association determines relationships between attributes in a database that often can be correlated as a result of causality; and -
Summarization generalizes task-specific data into a data cube that can create graphical representations. While several organizations (IBM, Oracle, Silicon Graphics, Angoss, SAS, and SPSS) offer data-mining technologies, the SAS Enterprise model is provided, herein, to show the technology interface (Figure 3). The SAS system supports a myriad of mining models, including decision trees, regression, and neutral networks. Figure 3: The SAS Enterprise data-mining technology (http://www.sas.com/products/miner/index.html).
Once mined, the data offers its owners knowledge regarding fraud, profiling, retention, and profit analyses. In general, these methods provide organizations using on-line analytical processing (OLAP) with a multidimensional, logical view of critical data. Thus, strategic knowledge can be derived using forecasting, trend analyses, and statistical analyses. For health care organizations, in particular, data-mining solutions can provide payors, hospitals, alternative delivery systems, clinics, physicians, and home health agencies with more succinct, meaningful answers to issues, such as: -
(In)effective methods of patient care; -
Local and regional health statistics; -
Cost analyses by segments of a population, provider plan, or demographic group; -
Drug subscription, treatment, utilization, etc.; -
Genetic treatment, medical research, and discovery; and -
(In)effective methods for mining "text-based" clinical data. As Figure 2 suggested, regional markets in Boxes 1 and 4 are best suited for these implementations. Moreover, Box 1 (Highly Competitive Medical Markets) is likely to serve as an ideal environment given the presence of larger number of payor plans and enrollees thereby resting on the notion that CHIN participants are in need of vital data analyses on cost effectiveness, profitability, treatment, resource utilization, etc. In noncompetitive environments (Box 4), the health information network fosters a coupling on dispersed groups where competition and economic dimensions are not the primary drivers of the implementation. To this end, CHIN technologies offer the industry the ability to better mine larger sets of data to accomplish four critical analyses: -
Fraud Detection Of particular interest to health care providers, this application features provider and patient profiling along with behavior and resource utilization, charting tools, and detection functionality. Data mining via CHIN technologies permits continuous monitoring on the above, yet these analyses are performed on a broader scale (i.e., local, state, and regional levels). Moreover, similar to fraud detection among credit card agencies, CHIN constituents, particularly government agencies, can mine data to discover (mis)uses of medical benefits, monetary losses, and legal infractions. -
Profitability Analysis Figure 1 and the material that follows clearly points out the criticality of the implementation process. As noted in Table 2, every CHIN participant organization was significantly concerned about the economic dimensions associated with investing in the network technologies in question. While the CHIN technology enables larger data sets to analyzed, its users can conduct additional regional analyses by metropolitan areas, counties, states, and districts. Effective health care plans, services, care delivery plans, and even those "profit generating" physicians - can be determined. Ineffective or non-profitable services can then be eliminated based on the more global analyses generated from data-mining technologies. -
Patient Profiling By using psychographic data, CHIN research members can gain amore extensive examination of patient behavior to uncover treatment and care service utilization patterns. For example, mined data could profile patients by physicians visited and medical tests ordered, drug treatment, gender, ethnicity, age, episode of illnesses, just to name a few. -
Retention Management Customer relationship management is linked to the notion of retention management. Ideally, health care payors would like to retain their existing customer bases while attracting and converting potential customers to enrollees. Data mining via the CHIN facilitates cross-selling and electronic marketing services. While the case can be made for preserving patient members, health care groups must also think in terms of "retaining" physician members as well. Thus, identifying services critical to participating physician groups can be just as fundamental. | | Brought to you by Team-Fly | | | |