P. Too Much Unplanned Maintenance Overview Sometimes a process breaks down at an unacceptably high rate, causing lost capacity, impacted delivery times, or just plain expensive maintenance. Clearly there are two elements here: Frequency of failure Duration of failure They combine to form a total cost of failure made up of (at least) Missed deliveries (lost revenue and possible loss of future revenue) High inventory Direct maintenance costs (parts, labor, etc.) Additional equipment kept to alleviate the problem (extra capacity or parts) Production defects (scrap and rework) attributable to breakdowns Lower reliability product Examples Industrial. Plant/equipment breakdown, line breakdown Healthcare. Information systems equipment failure (servers, systems), medical equipment breakdown (scanners, test equipment) Service/Transactional. Server breakdown, equipment breakdown (locomotives, tractor units, tracking systems, etc.) Measuring Performance If the failure rate were very high, it would be possible to count actual failures in a given period (breakdowns per month). More than likely, the failures per month is small and thus a better metric is the Mean Time Between Failures (MTBF). If duration of downtime were the key issue, measuring Total Downtime Due to Unplanned Maintenance would be a good metric. It will also be a good idea to roll this all back up into one metric of lost monies (revenue or profit dollars). Tool Approach The approach here would be to quickly determine what the best metric to drive the project would be: or 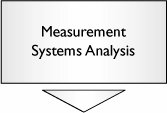 | For both measures, we will need to look at the validity of the metric; a sound operational definition and consistent measure versus a detailed investigation of Gage R&R will suffice. For more details see "MSAValidity" in Chapter 7, "Tools." | 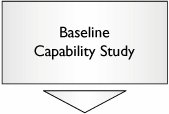 | Take a baseline measure of both metrics for a one-month period. Historical data will probably be good here for number of incidents, but might be a bit sketchy for duration of downtime. If it is not available, use whatever data is available on hand to make the call between the two metrics and set up a data collection to confirm the choice (i.e., continue on in parallel). If plenty of sound data is available, using a year's worth will be more than enough. It will also be necessary for each breakdown to get a cause of failure. This will be used later in the roadmap. |
From the Capability data, it should be apparent whether the issue is one of the downtime being the major issue, the number of incidents, or both. 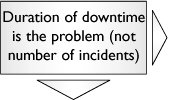 | If duration of downtime due to unplanned maintenance is the problem, the objective would be to reduce the time taken to bring the process back online after it is down. This is one of the tools in Total Productive Maintenance (TPM) and is known as Breakdown Maintenance. Go to Section N in this chapter to resolve this problem. | 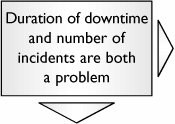 | If both issues prevail, the first thing would be to reduce the time taken to bring the process back online after it is down. This is one of the tools in Total Productive Maintenance (TPM) and is known as Breakdown Maintenance. Go to Section N in this chapter to resolve this problem and then return to this roadmap to reduce the number of incidents. |
At this point, either the time taken to bring the process back online has been minimized, or it was never a problem in the first place. The focus from this point forward is on what is causing it to go down in the first place. We would still work by our original metric (either time or number of incidents): 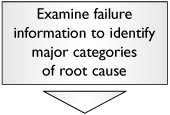 | Processes tend to break down based on a limited number of reasons. Simple examination of historical failure data, coupled with brainstorming with the maintenance group, will identify the finite number of clusters of failure types. | 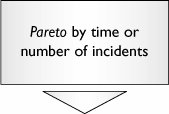 | Take the data used in the baseline Capability Study and determine the primary causes of failure using a Pareto Diagram. It is common for a few root causes to be generating the vast majority of breakdowns. From this point forward, the focus would be on the top 70%80% of failures (perhaps two to three reasons). |
The roadmap from here is based on the equation Y=f(X1, X2,..., Xn), where Y is either the Number of Incidents of Unplanned Maintenance or the Total Time Lost Due to Unplanned Maintenance. Throughout the following roadmap, the focus will only be on the process steps that break down, not the whole process. The reason for this is twofold: 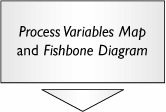 | A combined use of tools tends to work well here. Both tools are used to identify all the input variables (Xs) that could cause breakdown. Use the Process Variables Map first and then use a second pass of building a Fishbone Diagram for each process step to ensure absolutely all the Xs have been identified. Remember: Only list Xs for steps where the breakdown occurs. Any obviously problematic uncontrolled Xs should be added directly to the Process Failure Mode and Effects Analysis (FMEA). | 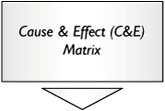 | The Xs generated by the Process Variables Map/Fishbone Diagram combination are transferred directly into the C&E Matrix. The Team uses its existing knowledge of the process through the matrix to eliminate the Xs that probably don't cause breakdown. At this point, there are usually just a few process steps being examined, so a single Phase C&E Matrix will suffice, but if for some reason many steps are involved, consider a three-phase C&E Matrix as follows: Phase 1 List the process steps (not the Xs) as the items to be prioritized in the C&E Matrix. Reduce the number of steps based on the effect of the steps as a whole on breakdowns. Phase 2 For the reduced number of steps, enter the Xs for only those steps into a second C&E Matrix and use this matrix to reduce the Xs to a manageable number. Phase 3 Make a quick check on Xs from the steps eliminated in Phase 1 to ensure that no obviously vital Xs have been eliminated. The Ys used for the C&E Matrix would be the primary failure types with the importance rating relating to the frequency of occurrence of that type. | 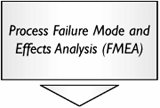 | The reduced set of Xs from the C&E Matrix is entered into the FMEA. This tool will narrow them down further, along with generating a set of action items to eliminate or reduce high-risk process areas. This is as far as the Team can proceed without detailed process data on the Xs. The FMEA is the primary tool to manage the obvious Quick Hit changes to the process that will eliminate special causes of breakdown. At this point, the problem might be reduced enough to proceed to the Control tools in Chapter 5. If not, continue down this roadmap. |
The project is now in the interesting position that the Belt should set up a longer-term data collection in the form of a Multi-Vari Study. During this study, the Belt should monitor data collection but is considered to be freed up enough to work on other things/project work. The Multi-Vari Study will be conducted on as many parallel lines as is deemed sensible to gather enough information. It would run for at least four months, although it would be useful to continue even longer to gain even more insight, especially if the MTBF is greater than seven days. 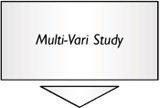 | The reduced set of Xs from the FMEA is carried over into this array of tools along with the Y being MTBF. Statistical tools applied to actual process data will help answer the questions: Which Xs (probably) affect the MTBF? Which Xs (probably) don't affect the MTBF? How much variation in the MTBF is explained by the Xs investigated? The word probably is used because this is statistics and hence there is a degree of confidence associated with every inference made. This tool will narrow the Xs down to the few key Xs that (probably) drive most of the variation in MTBF. |
At this point it is usually best to move straight to the Control tools in Chapter 5. It is uncommon in process reliability (versus product reliability) projects to undertake Designed Experiments, but is possible. To do this, the Xs from the Multi-Vari Study would be taken through the roadmap of Screening DOE, Characterizing DOE, and Optimization DOE. This is an interesting philosophical area for debate, but will not be addressed further here. |